Deep Learning for Automatic Diagnosis and Morphologic Characterization of Malignant Biliary Strictures Using Digital Cholangioscopy: A Multicentric Study
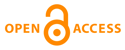
Autores da FMUP
Participantes de fora da FMUP
- Saraiva, MM
- Ribeiro, T
- Gonzalez Haba, M
- Castillo, BA
- Ferreira, J.
- Boas, FV
- Afonso, J
- Mendes, F
- Martins, M
- Cardoso, P
- Pereira, P
Unidades de investigação
Abstract
Simple Summary Diagnosis and characterization of biliary strictures is challenging, even after the introduction of digital single-operator cholangioscopy (D-SOC). The endoscopist's visual impression has a suboptimal accuracy and there is a significant interobserver variability. Artificial intelligence tools for image analysis have presented important contributions in several fields of gastroenterology. Convolutional neural networks are highly efficient multi-layered deep neural networks for image analysis, with great results in several fields of medicine. Nevertheless, the role of these deep learning models in digital cholangioscopy is still in a premature phase. With this bicentric international study, the authors aimed to create a deep learning-based algorithm for digital cholangioscopy capable of distinguishing benign from malignant biliary lesions. The present model accurately detected malignant biliary lesions with an image processing rate that favors its clinical applicability. The authors believe that the use of an AI-based model may change the landscape in the digital cholangioscopy diagnostic yield.Abstract Digital single-operator cholangioscopy (D-SOC) has enhanced the ability to diagnose indeterminate biliary strictures (BSs). Pilot studies using artificial intelligence (AI) models in D-SOC demonstrated promising results. Our group aimed to develop a convolutional neural network (CNN) for the identification and morphological characterization of malignant BSs in D-SOC. A total of 84,994 images from 129 D-SOC exams in two centers (Portugal and Spain) were used for developing the CNN. Each image was categorized as either a normal/benign finding or as malignant lesion (the latter dependent on histopathological results). Additionally, the CNN was evaluated for the detection of morphologic features, including tumor vessels and papillary projections. The complete dataset was divided into training and validation datasets. The model was evaluated through its sensitivity, specificity, positive and negative predictive values, accuracy and area under the receiver-operating characteristic and precision-recall curves (AUROC and AUPRC, respectively). The model achieved a 82.9% overall accuracy, 83.5% sensitivity and 82.4% specificity, with an AUROC and AUPRC of 0.92 and 0.93, respectively. The developed CNN successfully distinguished benign findings from malignant BSs. The development and application of AI tools to D-SOC has the potential to significantly augment the diagnostic yield of this exam for identifying malignant strictures.
Dados da publicação
- ISSN/ISSNe:
- 2072-6694, 2072-6694
- Tipo:
- Article
- Páginas:
- 4827-
- Link para outro recurso:
- www.scopus.com
Cancers Multidisciplinary Digital Publishing Institute (MDPI)
Citações Recebidas na Scopus: 2
Documentos
- Não há documentos
Filiações
Keywords
- cholangioscopy; artificial intelligence; biliary strictures
Financiamento
Proyectos asociados
The contribution of endoscopic ultrasound and biomarkers in the management of pancreatic adenocarcinoma and its precursor lesions.
Investigador Principal: Manuel Guilherme Gonçalves Macedo
Estudo Clínico Académico . 2023
Noninvasive serum biomarkers of portal hypertension in liver cirrhosis
Investigador Principal: Manuel Guilherme Gonçalves Macedo
Estudo Clínico Académico . 2023
Otimização do rendimento da colangiopancreatografia retrógrada endoscópica na avaliação das estenoses pancreato-biliares indeterminadas
Investigador Principal: Manuel Guilherme Gonçalves Macedo
Estudo Clínico Académico . 2023
Endoscopic Treatment Of Upper Gastrointestinal Postsurgical Leaks
Investigador Principal: Manuel Guilherme Gonçalves Macedo
Estudo Clínico Académico . 2023
Citar a publicação
Saraiva MM,Ribeiro T,Gonzalez M,Castillo BA,Ferreira J,Boas FV,Afonso J,Mendes F,Martins M,Cardoso P,Pereira P,Macedo G. Deep Learning for Automatic Diagnosis and Morphologic Characterization of Malignant Biliary Strictures Using Digital Cholangioscopy: A Multicentric Study. Cancers. 2023. 15. (19):p. 4827-4827. IF:5,200. (2).