Machine learning methods to predict attrition in a population-based cohort of very preterm infants
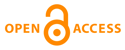
Autores da FMUP
Participantes de fora da FMUP
- Teixeira, R
- Rodrigues, C
- Moreira, C
- Camacho, R.
Unidades de investigação
Abstract
The timely identification of cohort participants at higher risk for attrition is important to earlier interventions and efficient use of research resources. Machine learning may have advantages over the conventional approaches to improve discrimination by analysing complex interactions among predictors. We developed predictive models of attrition applying a conventional regression model and different machine learning methods. A total of 542 very preterm (< 32 gestational weeks) infants born in Portugal as part of the European Effective Perinatal Intensive Care in Europe (EPICE) cohort were included. We tested a model with a fixed number of predictors (Baseline) and a second with a dynamic number of variables added from each follow-up (Incremental). Eight classification methods were applied: AdaBoost, Artificial Neural Networks, Functional Trees, J48, J48Consolidated, K-Nearest Neighbours, Random Forest and Logistic Regression. Performance was compared using AUC- PR (Area Under the Curve-Precision Recall), Accuracy, Sensitivity and F-measure. Attrition at the four follow-ups were, respectively: 16%, 25%, 13% and 17%. Both models demonstrated good predictive performance, AUC-PR ranging between 69 and 94.1 in Baseline and from 72.5 to 97.1 in Incremental model. Of the whole set of methods, Random Forest presented the best performance at all follow-ups [AUC-PR1: 94.1 (2.0); AUC-PR2: 91.2 (1.2); AUC-PR3: 97.1 (1.0); AUC-PR4: 96.5 (1.7)]. Logistic Regression performed well below Random Forest. The top-ranked predictors were common for both models in all follow-ups: birthweight, gestational age, maternal age, and length of hospital stay. Random Forest presented the highest capacity for prediction and provided interpretable predictors. Researchers involved in cohorts can benefit from our robust models to prepare for and prevent loss to follow-up by directing efforts toward individuals at higher risk.
Dados da publicação
- ISSN/ISSNe:
- 2045-2322, 2045-2322
- Tipo:
- Article
- Páginas:
- -
Scientific Reports Nature Publishing Group
Citações Recebidas na Web of Science: 1
Documentos
- Não há documentos
Filiações
Keywords
- BIRTH-WEIGHT INFANTS; FOR-GESTATIONAL-AGE; YOUNG ADULTHOOD; MISSING DATA; PARTICIPATION; PREVALENCE; OUTCOMES; EUROPE
Financiamento
Proyectos asociados
Preexposure prophylaxis for HIV prevention among men who have sex with men: understanding eligibility and early uptake
Investigador Principal: José Henrique Dias Pinto de Barros
Estudo Clínico Académico . 2021
Eficácia de intervenções educacionais comunitárias em nutrição e WASH (Water, Sanitation and Hygiene) / Malária na diminuição da prevalência de anemia e malnutrição em crianças menores de 5 anos
Investigador Principal: José Henrique Dias Pinto de Barros
Estudo Clínico Académico . 2021
Estudo dos factores de risco cardiovascular numa população adulta da Província do Bengo, Angola
Investigador Principal: José Henrique Dias Pinto de Barros
Estudo Clínico Académico . 2019
Citar a publicação
Teixeira R,Rodrigues C,Moreira C,Barros H,Camacho R. Machine learning methods to predict attrition in a population-based cohort of very preterm infants. Sci. Rep. 2022. 12. (1):10587. IF:4,600. (2).