Identification of avoidable patients at triage in a Paediatric Emergency Department: a decision support system using predictive analytics
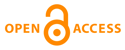
Unidades de investigação
Abstract
BackgroundCrowding has been a longstanding issue in emergency departments. To address this, a fast-track system for avoidable patients is being implemented in the Paediatric Emergency Department where our study is conducted. Our goal is to develop an optimized Decision Support System that helps in directing patients to this fast track. We evaluated various Machine Learning models, focusing on a balance between complexity, predictive performance, and interpretability.MethodsThis is a retrospective study considering all visits to a university-affiliated metropolitan hospital's PED between 2014 and 2019. Using information available at the time of triage, we trained several models to predict whether a visit is avoidable and should be directed to a fast-track area.ResultsA total of 507,708 visits to the PED were used in the training and testing of the models. Regarding the outcome, 41.6% of the visits were considered avoidable. Except for the classification made by triage rules, i.e. considering levels 1,2, and 3 as non-avoidable and 4 and 5 as avoidable, all models had similar results in model's evaluation metrics, e.g. Area Under the Curve ranging from 74% to 80%.ConclusionsRegarding predictive performance, the pruned decision tree had evaluation metrics results that were comparable to the other ML models. Furthermore, it offers a low complexity and easy to implement solution. When considering interpretability, a paramount requisite in healthcare since it relates to the trustworthiness and transparency of the system, the pruned decision tree excels.Overall, this paper contributes to the growing body of research on the use of machine learning in healthcare. It highlights practical benefits for patients and healthcare systems of the use ML-based DSS in emergency medicine. Moreover, the obtained results can potentially help to design patients' flow management strategies in PED settings, which has been sought as a solution for addressing the long-standing problem of overcrowding.ConclusionsRegarding predictive performance, the pruned decision tree had evaluation metrics results that were comparable to the other ML models. Furthermore, it offers a low complexity and easy to implement solution. When considering interpretability, a paramount requisite in healthcare since it relates to the trustworthiness and transparency of the system, the pruned decision tree excels.Overall, this paper contributes to the growing body of research on the use of machine learning in healthcare. It highlights practical benefits for patients and healthcare systems of the use ML-based DSS in emergency medicine. Moreover, the obtained results can potentially help to design patients' flow management strategies in PED settings, which has been sought as a solution for addressing the long-standing problem of overcrowding.
Dados da publicação
- ISSN/ISSNe:
- 1471-227X,
- Tipo:
- Article
- Páginas:
- -
- Link para outro recurso:
- www.scopus.com
BMC Emergency Medicine BioMed Central Ltd.
Documentos
- Não há documentos
Filiações
Filiações não disponíveis
Keywords
- Paediatrics; Triage; Emergency Services; AI; Machine Learning; Fast Track; Management
Proyectos asociados
Stimulate continous monitoring in personal and physical health.
Investigador Principal: José Alberto da Silva Freitas
Estudo Observacional Académico (INNO4HEALTH) . FCT . 2021
Estudos de avaliação de exequibilidade, usabilidade e utilização de uma app para telemóvel para gestão da diabetes tipo 2.
Investigador Principal: José Alberto da Silva Freitas
Estudo Observacional Académico (FoodFriend) . FCT . 2022
Portuguese Public Hospitals Financial Performance between 2014-2020
Investigador Principal: José Alberto da Silva Freitas
Estudo Clínico Académico (Financial Performance) . 2023
Tendências nas Hospitalizações por Insuficiência Cardíaca durante um Período de Dezasseis Anos: Dados de Abrangência Nacional para Portugal
Investigador Principal: José Alberto da Silva Freitas
Estudo Clínico Académico (Hospitalizações IC) . 2022
The use of secondary data in Mental Health research
Investigador Principal: José Alberto da Silva Freitas
Estudo Clínico Académico . 2023
Health priorities in the European Union - a novel framework
Investigador Principal: José Alberto da Silva Freitas
Estudo Clínico Académico . 2023
Healthcare Human Resources and Quality Indicators: Approaches to Strengthening Primary Care.
Investigador Principal: José Alberto da Silva Freitas
Estudo Clínico Académico . 2022
A machine learning-based approach to support the assessment of clinical coded data quality in the context of Diagnosis-Related Groups classification systems
Investigador Principal: José Alberto da Silva Freitas
Estudo Clínico Académico . 2020
Citar a publicação
Viana J,Souza J,Rocha R,Santos A,Freitas A. Identification of avoidable patients at triage in a Paediatric Emergency Department: a decision support system using predictive analytics. BMC Emerg. Med. 2024. 24. (1):149. IF:2,500. (2).