MedGAN: optimized generative adversarial network with graph convolutional networks for novel molecule design
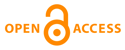
Unidades de investigação
Abstract
Generative Artificial Intelligence can be an important asset in the drug discovery process to meet the demand for novel medicines. This work outlines the optimization and fine-tuning steps of MedGAN, a deep learning model based on Wasserstein Generative Adversarial Networks and Graph Convolutional Networks, developed to generate new quinoline-scaffold molecules from complex molecular graphs, including hyperparameter adjustments and evaluations of drug-likeness attributes such as pharmacokinetics, toxicity, and synthetic accessibility. The best model was capable of generating 25% valid molecules, 62% fully connected, from which 92% were quinolines, 93% were novel, and 95% unique, preserving chirality, atom charge, and favorable drug-like properties while generating 4831 novel quinolines. These results provide valuable insights into how activation functions, optimizers, learning rates, neuron units, molecule size and constitution, and scaffold structure affect the performance of generative models and their potential to create new molecular structures, enhancing deep learning applications in computational drug design.
Dados da publicação
- ISSN/ISSNe:
- 2045-2322, 2045-2322
- Tipo:
- Article
- Páginas:
- -
- PubMed:
- 38216614
- Link para outro recurso:
- www.scopus.com
Scientific Reports Nature Publishing Group
Citações Recebidas na Web of Science: 5
Citações Recebidas na Scopus: 8
Documentos
- Não há documentos
Filiações
Filiações não disponíveis
Keywords
- DRUG DISCOVERY
Projetos associados
Impact of EU label changes and regulatory communication on SARS-CoV-2 adenovirus vector vaccines in context of thrombosis with thrombocytopenia syndrome (TTS): risk awareness and adherence.
Investigador Principal: Fernanda Inês de Carvalho Pereira Ribeiro
Estudo Observacional Académco (RiskAwareTTS) . 2022